











|
Tutorial Sessions
Tutorial Chair:
Dr Pierre Dersin, Alstom Transport, St-Ouen, France
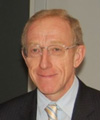
Tutorial Vice Chair:
Dr Sony Mathew, Schlumberger, Houston, Texas, USA
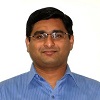
___________________________________________________________________________________________
Tutorial 1: Monitoring of Safran Aircraft Engines Fleet
Instructors:
Marion Jedruszek , SAFRAN Aircraft Engines, France
Josselin Coupard , SAFRAN Aircraft Engines, France
Tutorial Abstract:
More than 40 years ago SAFRAN Aircraft Engines and General Electric built the CFM International Joint Venture to produce CFM56, the best seller engine for medium range aircrafts (Airbus A320 series and Boeing B737 series). This engine was already equipped with PHM applications among which online crack detection in bearings and performance monitoring. Nowadays, this engine is progressively replaced by the new LEAP version which embed lot more analytic tools thanks to the improvement of computing power and data transmission. SAFRAN also works on other applications: military engines for the French Rafale aircraft and civil applications like the PowerJet Sam146 for the Sukhoi aircraft with NPO Saturn, a Russian engine manufacturer, and more recently the smaller Silvercrest engine dedicated to business aircrafts F5X Dassault and future Cessna Citation Hemisphere.
Our PHM system technology relies on two major components, online embedded computations and ground applications, but also on our extended maintenance knowledge databases. The online component manages sensors and most high level indicator computations, then it broadcasts this data to the ground with different technologies, during the flight for low frequency snapshots and high frequency full flight data at the airport gate. Most of the system intelligence is implemented on the ground application. A main part is based on our technology skill inserted in the software as physical models, maintenance manuals and decision schemes. But since the last ten years new intelligent algorithms enter the decision process, like automatic detection of abnormalities based on statistical observations, digital classification of engine states and identification of early symptoms of potential degradation. Those new mathematical tools extensively use our main maintenance knowledge to calibrate and evaluate the quality of the algorithms.
This tutorial starts by a reminder of the need for health-monitoring as an optimization tool for diminishing the global earned cost of an aircraft engine but also a help to improve the image of our customer business. Then we will enter into the concept of an aircraft propulsion system, list the main functions to monitor and see what the available data are and how to manage them. The embedded system will be detailed with all constraints related to an aircraft system. The ground system, including the trouble shooting and decision process will be addressed using two red line examples: the monitoring of the oil system and the evolution of the exhaust gas temperature which is a good wear indicator for a turbofan.
Presenters’ Biographies:
Jérôme Lacaille is an emeritus expert in algorithms for Safran international aeronautic group. He joined the Snecma Company in 2007 with responsibility for developing a health monitoring solution for jet engines. Jérôme has a PhD from the Ecole Normale Supérieure (France) in Mathematics. He has held several positions including scientific consultant and professor. He has also co-founded the Miriad Technologies Company, entered the semiconductor business taking in charge the direction of the Innovation Department for Si Automation (Montpellier - France) and PDF Solutions (San Jose - CA). He developed specific mathematic algorithms that were integrated in industrial processes. Over the course of his work, Jérôme has published several papers on integrating data analysis into industry infrastructure, including neural methodologies and stochastic modeling as well as some industrial patented applications.
___________________________________________________________________________________________
Tutorial 2: Survival Analysis with Complex Covariates: A Model-Based Clustering Preprocessing Step
Instructors:
Dr. Christophe Biernacki, Professor, France and INRIA-Model Laboratory, University of Lille, France
Dr. Vincent Vandewalle, France and INRIA-Model Laboratory, University of Lille, France
Tutorial Abstract:
Many covariates are now available through sensors in the industrial context, and are expected to be related to the survival analysis target. Such covariates are often complex, what has to be understood as a possible mix between continuous, categorical, even functional over time, variables with the possibility to contain missing or uncertain values. A natural question in survival analysis is to design in both flexible and easy way an hazard function related to these potentially complex covariates, while preserving the opportunity to benefit from classical hazard functions.
In this tutorial, we will propose to decompose this unknown targeted hazard function into two complementary parts. The first one can be any classical user hazard function conditional on a latent categorical variable. The second one is the distribution of this latent variable conditionally to the complex covariates. The way to combine both parts is to sum their product over the latent variable (marginal distribution), leading to the final targeted hazard function.
The key to perform this approach is to focus on the latent variable definition which can be obtained with a model based clustering approach dedicated to complex covariates. In this tutorial we will give a selected review of recent methodologies dedicated to clustering. Beyond methodology, we will describe in depth some related software to perform previous clustering methods. Some case studies will be also provided in an industrial context. At the end of the talk the practitioner will be thus able to perform such clustering method to use it finally with its own hazard function.
Presenter’s Biography:
Christophe Biernacki is a Professor of Statistics, scientific leader of the Inria Modal team. His research focuses on model-based classification and clustering. He is a specialist of dealing with complex data including a mix of continuous, categorical, functional, uncertain and missing data. In this context, he co-developed the MIXMOD and MIXTCOMP software for mixture models.
Vincent Vandewalle is an Assistant Professor of Statistics, he is member of the Inria Modal team. His research focuses on model-based clustering and classification. He is a specialist of dealing with complex data including a mix of continuous, categorical, functional, uncertain and missing data.
___________________________________________________________________________________________
Tutorial 3: Physics-of-Failure Algorithms for Prognostics of Electronics
Instructor:
Dr. Michael Osterman, University of Maryland, USA
Tutorial Abstract:
Reliability is the ability of a product to perform as intended (i.e., without failure and within specified performance limits) for a specified time, in its life cycle application environment. The physics-of-failure (PoF) approach to reliability utilizes knowledge of the life-cycle load profile, product architecture, and material properties to identify potential failure mechanisms and to reduce product failures through robust design, manufacturing, and product verification practices. PoF-based product realization methods incorporate reliability into the development process by providing a scientific basis for estimating product life under life cycle conditions. Estimating the product life cycle conditions is a critical component of PoF and provides the impetus for prognostics.
This tutorial introduces classical reliability concepts and relates the concepts to the PoF approach to reliability and prognostics. It will present PoF-based failure mechanisms and their models as well as approaches for implementing PoF-based prognostics. The focus for this presentation will be on electronic equipment, and it includes the follwoing four parts: 1) introduction, 2) level failure mechanism and models, 3) PoF simulation assisted reliability assessment, and 4) PoF-based prognostics.
Presenter’s Biography:
Michael Osterman (Ph.D., Engineering Mechanics, University of Maryland, College Park) is a Senior Research Scientist and the Director of the CALCE Electronic Products and Systems Consortium at the University of Maryland. He is involved in the development of simulation-assisted reliability assessment software and simulation approaches for estimating the time-to-failure of electronic hardware under test and field conditions. He has written various book chapters and over 90 articles in the area of electronics packaging. He is a senior member of IEEE and a member of ASME, IMAPS, and SMTA.
___________________________________________________________________________________________
Tutorial 4: A Tutorial on Structural Health Monitoring
Instructor: Dr. Charles R. Farrar, The Engineering Institute, Los Alamos National Laboratory, USA
Tutorial Abstract:
The process of implementing a damage detection strategy for aerospace, civil and mechanical engineering infrastructure is referred to as structural health monitoring (SHM). The SHM process compliments traditional nondestructive evaluation by extending these concepts to online, in situ system monitoring on a more global scale. For long term SHM, the output of this process is periodically updated information regarding the ability of the structure to perform its intended function in light of the inevitable aging and degradation resulting from operational environments. After more extreme events, SHM is used for rapid condition screening and aims to provide, in near real time, reliable information regarding the integrity of the structure.
This tutorial will begin by briefly summarize the historical developments of SHM technology. Next, the current state of the art is summarized where the SHM problem is described in terms of a statistical pattern recognition paradigm. In this paradigm, the SHM process can be broken down into four parts: (1) Operational Evaluation, (2) Data Acquisition and Cleansing, (3) Feature Selection and Extraction, and (4) Statistical Model Development for Feature Discrimination. The tutorial will provide overviews of each portion of this process and will present examples of different approaches that can be taken to accomplish these necessary tasks using examples from aerospace, civil and mechanical engineering systems. In addition, outstanding research challenges for each portion of the paradigm will be discussed along with more global system level challenges. Next, a discussion of fundamental axioms for SHM that form a foundation for developing a SHM technology will be presented. The tutorial will conclude with a brief summary of some emerging technologies that will potentially impact future SHM development and deployment.
Presenter’s Biography:
Charles R. (Chuck) Farrar (Ph.D., PE) has over 30 years of experience at Los Alamos National Laboratory (LANL). He is currently the director of LANL’s Engineering Institute, a research and education collaboration between LANL and the Univ. of California San Diego’s (UCSD) Jacobs School of Engineering. His research interests focus on developing integrated hardware and software solutions to structural health monitoring (SHM) problems and the development of damage prognosis technology. The results of this research have been documented in over 400 publications (refereed journal articles, book chapters, conference papers, Los Alamos reports), numerous keynote lectures at international conferences, and most recently in a book co-authored with Prof. Keith Worden entitled Structural Health Monitoring A Machine Learning Perspective. His work has been recognized at LANL through his reception of the inaugural Los Alamos Fellows Prize for Technical Leadership and by the SHM community through the reception of the inaugural Lifetime Achievement Award in Structural Health Monitoring. Each year he teaches a graduate course on SHM for UCSD. Additional professional activities include the development of a structural health monitoring short course that has been offered more than 35 times to industry and government agencies in Asia, Australia, Europe and the U.S. In 2012 he was elected as a Fellow of Los Alamos National Laboratory. He is also a Fellow of the American Society of Mechanical Engineers and American Society of Civil Engineers.
___________________________________________________________________________________________
Tutorial 5: Deep Learning, Computational Intelligence and Health Monitoring of Machines
Instructor:
Dr. Nishchal K. Verma, Associate Professer, IIT Kanpur, India
Tutorial Abstract:
Deep Learning (DL) deals with a set of algorithms developed to achieve deeper intuitions and intricate structures within data. DL framework comprises of multiple layers of nonlinear processing units which are trained over a large set of case specific data. DL algorithms have revolutionized representation learning in unsupervised or semi-supervised manner, thereby improving state-of-art in various fields of computational Intelligence such as pattern recognition and detection and big data analytics, etc. Computational Intelligence (CI) involves a set of biological and linguistic computational tools and methodologies to address complex and challenging problems to which traditional approaches may not be very effective. Major constituents of CI are neural networks, fuzzy systems, and hybrid intelligent systems. For Health Monitoring of Machines the early recognition of faults saves industry from heavy losses occurring due to untimely machine breakdowns. In this tutorial, DL and CI based algorithms will be discussed for Health Monitoring of Machines.
Presenter’s Biography:
Nishchal K. Verma is an Associate Professor with Department of Electrical Engineering, IIT Kanpur, India and recipient of Devendra Shukla Young Faculty Research Fellowship from IIT Kanpur for 2013-16. He is an IETE Fellow, IEEE Senior Member, and was the Founding Chairman of IEEE UP Section Computational Intelligence Society Chapter from 2013 to 2015. His research interests include Deep Learning, Computational Intelligence, Big data, Internet of Things, Intelligent Data Mining Algorithms, Diagnosis and Prognosis for Health Management, Computer Vision, and Cyber Physical Systems. He has authored/co-authored more than 160 research papers in reputed national and international journals and conferences. Dr. Verma is the Editor of IETE Technical Review, an Associate Editor of the IEEE Computational Intelligence Magazine, Transactions of the Institute of Measurement and Control, and Editorial Board Member for several reputed national and international journals and conferences.
Return to conference program
|